Speakers
Mon, Dec 14 | Tue, Dec 15 | Wed, Dec 16 | |
08:30 – 09:00 | Registration | ||
09:00 – 10:30 | Janusz Konrad (Boston University) | Shi Boxin (Peking University) | Ling Guan (Ryerson University) |
10:30 – 11:00 | Break | Break | |
11:00 – 12:30 | Lin Weisi (Nanyang Technological Univeristy) | Shi Boxin (Peking University) | Dr. Xiao-Ping (Steven) Zhang (Ryerson University) |
12:30 – 14:00 | Lunch Break | Lunch Break | END |
14:00 – 15:30 | Lin Weisi (Nanyang Technological Univeristy) | Cheong Ngai-Man (Singapore University of Technology and Design) | |
15:30 – 16:00 | Break | Break | |
16:00 – 17:30 | Cheung Ngai-Man (Singapore University of Technology and Design) | Andrea Cavallaro (Queen Mary University of London) | |
END |
All timings are given in Singapore time (GMT+8).
IEEE Signal Processing Society Distinguished Lectures (supported by IEEE SPS Singapore Chapter)
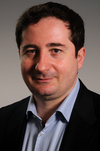
Andrea Cavallaro
Professor
Queen Mary University of London
Title : Adversarial attacks on image classifiers
Synopsis : Images we share online reveal information about personal choices and preferences, which can be inferred by classifiers. To prevent privacy violations and to protect the visual content from unwanted automatic inferences, I discuss how to exploit the vulnerability of classifiers to adversarial attacks to craft perturbations that maintain (and even improve) image quality. As adversarial perturbations designed to protect visual content against classifiers may be ineffective against classifiers that were not seen during the generation of the perturbation or against defences that use re-quantization or image compression, I will discuss how to craft perturbations based on randomised ensembles to make them robust to defences, on image semantics to selectively modify colours within chosen ranges that are perceived as natural by humans, and on perturbations that enhance image details.
Biodata :Andrea Cavallaro is Professor of Multimedia Signal Processing and the founding Director of the Centre for Intelligent Sensing at Queen Mary University of London, UK. He is Fellow of the International Association for Pattern Recognition (IAPR) and Turing Fellow at the Alan Turing Institute, the UK National Institute for Data Science and Artificial Intelligence. He is Editor-in-Chief of Signal Processing: Image Communication; Chair of the IEEE Image, Video, and Multidimensional Signal Processing Technical Committee; an IEEE Signal Processing Society Distinguished Lecturer; an elected member of the IEEE Video Signal Processing and Communication Technical Committee; and a Senior Area Editor for the IEEE Transactions on Image Processing. Prof. Cavallaro received his Ph.D. in Electrical Engineering from the Swiss Federal Institute of Technology (EPFL), Lausanne, in 2002. He was a Research Fellow with British Telecommunications (BT) in 2004/2005 and was awarded the Royal Academy of Engineering Teaching Prize in 2007; three student paper awards on target tracking and perceptually sensitive coding at IEEE ICASSP in 2005, 2007 and 2009; and the best paper award at IEEE AVSS 2009. He is a past Area Editor for the IEEE Signal Processing Magazine (2012-2014) and past Associate Editor for the IEEE Transactions on Image Processing (2011-2015), IEEE Transactions on Signal Processing (2009-2011), IEEE Transactions on Multimedia (2009-2010), IEEE Signal Processing Magazine (2008-2011) and IEEE Multimedia. He is a past elected member of the IEEE Multimedia Signal Processing Technical Committee and past chair of the Awards committee of the IEEE Signal Processing Society, Image, Video, and Multidimensional Signal Processing Technical Committee. Prof. Cavallaro has published over 270 journal and conference papers, one monograph on Video tracking (2011, Wiley) and three edited books: Multi-camera networks (2009, Elsevier); Analysis, retrieval and delivery of multimedia content (2012, Springer); and Intelligent multimedia surveillance (2013, Springer).

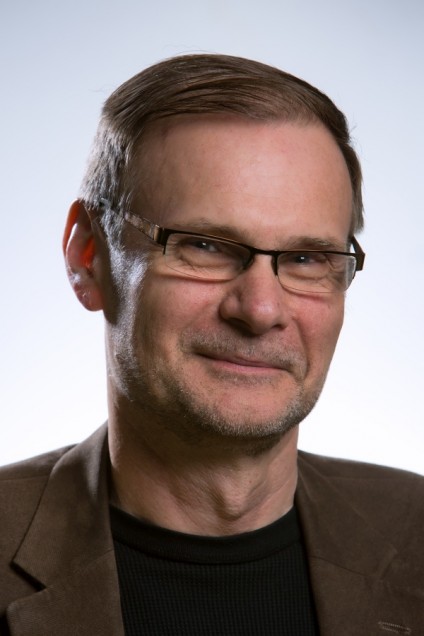
Janusz Konrad
Professor
Boston University
Title : Privacy-Preserving Localization and Recognition of Human Activities
Synopsis : Smart rooms, that respond to occupant behavior, will likely become common occurrence in our lifetimes. With advanced sensors, processors and algorithms, such rooms are expected to save energy and provide productivity as well as health benefits. Indoor localization of occupants and recognition of their activities are two key components of this vision. However, traditional camera-based systems may not be acceptable in privacy-sensitive scenarios since high-resolution images may reveal room details and occupant identity to eavesdroppers. I will first review traditional approaches to protecting occupant’s visual privacy, including reversible methods (e.g., data scrambling) and irreversible methods (e.g., optical and digital obfuscation of visual data). Then, I will describe computational solutions via resolution reduction recently developed at Boston University. In one approach, a small network of ceiling-mounted, single-pixel RGB sensors collects visual data from which occupant’s location is estimated by means of advanced algorithms. In another approach, extremely low resolution videos (e.g., 16 x 12 pixels) are used to recognize occupant’s activities. Since these algorithms are sensitive to illumination changes in the environment, I will offer one solution we developed that is based on active scene illumination.

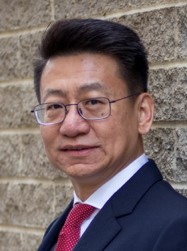
Xiao-Ping (Steven) Zhang
Professor
Ryerson University
Title : Signal Processing Path to Nobel Prize in Economics – Risk, Correlation, Causality and Predictive Analytics in Big Data
Synopsis : Economic data and financial markets are intriguing to researchers working on big data and quantitative models. With rapid growth and increasing access to data in digital form, finance, economics, and marketing data are poised to become one of the most important and tangible big data applications, owing not only to the relative clean organization and structure of the data but also to clear application objectives and market demands. However, data related economic and social science studies often have different viewpoints from signal processing (SP) and artificial intelligence (AI).
This talk intends to introduce some foundational concepts in finance/economics/marketing research, from signal and data processing point of view. Some of these ideas led to Nobel Prize in Economics. We explain the different focuses between economic and social science data analysis and physical signal processing, such as co-integration and causality analysis. For example, in most physical systems using signal processing and machine learning, the causality (input/output) relationship is often known and taken for granted, but it is generally not obvious/unknown in social and economic sciences. It is critical to discriminate causalities from spurious correlations in data. We also introduce the concept of predictive analytics from probabilistic point of view. We hope to inspire signal processing researchers to broaden their knowledge beyond their current areas of expertise and grasp some basics concepts and evaluation criteria in economics and social science fields.
Dr. Zhang is a Fellow of Canadian Academy of Engineering, a Fellow of IEEE, a registered Professional Engineer in Ontario, Canada, and a member of Beta Gamma Sigma Honor Society. He is the general Co-Chair for the IEEE International Conference on Acoustics, Speech, and Signal Processing, 2021. He is the general co-chair for 2017 GlobalSIP Symposium on Signal and Information Processing for Finance and Business, and the general co-chair for 2019 GlobalSIP Symposium on Signal, Information Processing and AI for Finance and Business. He is an elected Member of the ICME steering committee. He is the General Chair for the IEEE International Workshop on Multimedia Signal Processing, 2015. He is the Publicity Chair for the International Conference on Multimedia and Expo 2006, and the Program Chair for International Conference on Intelligent Computing in 2005 and 2010. He served as a Guest Editor for Multimedia Tools and Applications and the International Journal of Semantic Computing. He was a tutorial speaker at the 2011 ACM International Conference on Multimedia, the 2013 IEEE International Symposium on Circuits and Systems, the 2013 IEEE International Conference on Image Processing, the 2014 IEEE International Conference on Acoustics, Speech, and Signal Processing, the 2017 International Joint Conference on Neural Networks and the 2019 IEEE International Symposium on Circuits and Systems. He is a Senior Area Editor for the IEEE TRANSACTIONS ON SIGNAL PROCESSING and the IEEE TRANSACTIONS ON IMAGE PROCESSING. He was an Associate Editor for the IEEE TRANSACTIONS ON IMAGE PROCESSING, the IEEE TRANSACTIONS ON MULTIMEDIA, the IEEE TRANSACTIONS ON CIRCUITS AND SYSTEMS FOR VIDEO TECHNOLOGY, the IEEE TRANSACTIONS ON SIGNAL PROCESSING, and the IEEE SIGNAL PROCESSING LETTERS. He is elected Vice-Chair (Chair-elect) for Image, Video, and Multidimensional Signal Processing Technical Committee (IVMSP TC) of IEEE Signal Processing Society. He received 2020 Sarwan Sahota Ryerson Distinguished Scholar Award – the Ryerson University highest honor for scholarly, research and creative achievements. He is selected as IEEE Distinguished Lecturer for the term from January 2020 to December 2021 by IEEE Signal Processing Society.

VISVA 2020 Speakers
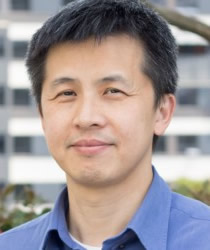
Cheung Ngai-Man
Professor
Singapore University of Technology and Design (SUTD)
Title : Deep Generative Model - Recent Advances and Applications
Synopsis : Recent years have seen remarkable advances in deep generative models such as auto-regressive networks, variational autoencoders (VAEs), generative adversarial networks (GANs). Deep generative models become effective approaches in learning high-dimensional, complex data distributions and creating novel samples and high-resolution images.
In this talk, I will first review fundamental ideas of deep generative models with focuses on GANs and important variants Wasserstein GANs. Then, I will discuss the latest advances in this area: Self-supervised GANs, training GANs with limited data, detection of GAN-generated images and Deepfakes, and recent GAN-based methods to reveal private training data from a trained model.

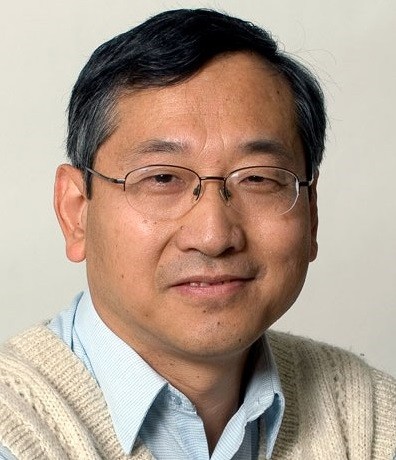
Ling Guan
Professor
Ryerson University
Title : Knowledge Discovery: When Statistical and Classical Methods Prevail?
Synopsis : Knowledge discovery has been playing a key role in machine learning for visual information processing and visualization. This talk starts with an overview on knowledge discovery and its processing pipeline, and then focus on detecting key-points for the extraction of quality features, and feature coding/transformation to generate effective (even optimal) information representation, two aspects in which statistical and classical machine learning methods possess a high probability to prevail.
For key-point detection, we introduce SCK, a universal key-point detector built upon the theory of sparse coding. SCK can handle any visual structures (blobs, corners, junctions, and more) and has been analytically proven to be invariant to changes in illumination, and spatial and rotational transformations. SCK’s superior performance has been justified by theoretical analysis and comparison with the well known hand-crafted detectors such as SIFT, Harris Corner, SFOP, etc., and state-of-the-art learningbased detectors. The code is recently being optimized to reach real-time or near real-time performance, a key requirement for a broad range of applications such as navigation systems in autonomous vehicles and computer guided brain and cardiac surgeries.
For feature coding/transformation, we have been studying a family of mathematically inspired methods which optimizes information representation in multimedia processing. It has been demonstrated that, combined with an elementary DNN structure such as AlexNet, this approach is compared favorably to some of the pure deep learning architecture with hundreds of layers, promising a simple, effective and academically relevant direction for machine learning research. Recent investigation shows that, like learning based methods, this approach is capable of incrementally accepting new data to adaptively update the learning model, thus laying the foundation for a robust and effective platform for big data analytics.

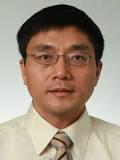
LIN Weisi
Professor
Nanyang Technological University
Title : Formulating Visual Significance & Sensitivity toward Big-data Analytics
Synopsis : Image processing, computer vision and other related AI tasks have to deal with big visual data, and therefore call for effective, efficient, and light-weight/green signal representations; to this end, evaluating visual significance and sensitivity enables differential treatments of visual signals (images and videos) throughout their life cycles: creation, compression, transmission, storage, reconstruction, and task performing, to tackle various big-data-induced difficulties for resources (e.g., bandwidth, memory, battery, computing power, device size, etc). In particular, visual attention (VA) models extract global spatial significance distributions, while just-noticeable difference (JND) ones provide local sensitivity thresholds. Such models can aim at systems and services for either humans or machines as final users (without human intervention). In this session, we will first concentrate on human-oriented VA and JND models, because the majority of existing related applications are still for human uses. We will introduce the research problems associated with VA and JND, as well as the relevant physiological and psychological ground. Afterward, we are to discuss the principles of the associated computational modelling and the advances in the area, including the bottom-up, top-down and hybrid approaches for VA, and subband-, pixel- and picture- based ones for JND, as well as the combination of VA and JND. Meaningful applications in perceptual quality assessment, image retargeting, visual search, image classification, person re-ID, style transfer, video coding, computer graphics, and target identification, as well as the relevant industrial deployment, are then highlighted. As an extension, machine-oriented modelling possibilities are to be explored, since machines are expected to make increasingly more decisions in this AI era. The talk will also present our opinions toward future R&D in other aspects (including effort to true multimedia that consists of audio, haptics, olfaction and gestation as well).
Biodata: Lin Weisi is an active researcher in intelligent image processing, perception-based signal modelling and assessment, video compression, and multimedia communication systems. He had been the Lab Head, Visual Processing, in Institute for Infocomm Research (I2R). He is a Professor and the Programme Director (Special Projects) in School of Computer Science and Engineering, Nanyang Technological University, where he also served as the Associate Chair (Graduate Studies) in 2013-2014. He is a Fellow of IEEE and IET, and an Honorary Fellow of Singapore Institute of Engineering Technologists. He has been awarded Highly Cited Researcher 2019 by Web of Science, and elected as a Distinguished Lecturer in both IEEE Circuits and Systems Society (2016-17) and Asia-Pacific Signal and Information Processing Association (2012-13), and given keynote/invited/tutorial/panel talks to 20+ international conferences during the past 10 years. He has been an Associate Editor for IEEE Trans. on Image Processing, IEEE Trans. on Circuits and Systems for Video Technology, IEEE Trans. on Multimedia, IEEE Signal Processing Letters, Quality and User Experience, and Journal of Visual Communication and Image Representation. He was also the Guest Editor for 7 special issues in international journals, and chaired the IEEE MMTC QoE Interest Group (2012-2014); he has been a Technical Program Chair for IEEE Int’l Conf. Multimedia and Expo (ICME 2013), International Workshop on Quality of Multimedia Experience (QoMEX 2014), International Packet Video Workshop (PV 2015), Pacific-Rim Conf. on Multimedia (PCM 2012) and IEEE Visual Communications and Image Processing (VCIP 2017). He believes that good theory is practical, and has delivered 10+ major systems and modules for industrial deployment with the technology developed.

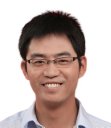
SHI Boxin
Professor
Peking University
Title : Complex Artifacts Removal from Contaminated Images
Synopsis : Artifacts are unavoidable when we capture images in the real world, which brings various distortion to negatively affect the image quality. There are two types of artifacts that are particularly complex in terms of formation, shapes and distribution: one is the reflection when capturing in front of the glass, the other one is the moire pattern when capturing in front of the screen. In this talk, I will introduce our latest progress in: 1) Data-driven single image reflection removal (90 min): The first benchmark dataset in this area, computational imaging based and deep learning based reflection removal solutions. 2) Reflection removal with recognizable features (45min): How to jointly perform image restoration and recognition by considering mutual benefits of optimizing low-level and high-level computer vision tasks. 3) Data-driven single-image moiré pattern removal (45 min): How to integrate special properties of the moiré pattern such as frequency distribution to effectively get rid of its contamination.
Biodata : Boxin Shi received the BE degree from the Beijing University of Posts and Telecommunications, the ME degree from Peking University, and the PhD degree from the University of Tokyo, in 2007, 2010, and 2013. He is currently a Boya Young Fellow Assistant Professor and Research Professor at Peking University, where he leads the Camera Intelligence group. He also serves as the Assistant Director at the Institute for Artificial Intelligence, Peking University, an Adjunct Professor at Beijing University of Posts and Telecommunications, and a Young Scientist at Beijing Academy of Artificial Intelligence. Before joining PKU, he did postdoctoral research with MIT Media Lab, Singapore University of Technology and Design, Nanyang Technological University from 2013 to 2016, and worked as a researcher in the National Institute of Advanced Industrial Science and Technology from 2016 to 2017. He won the Best Paper Runner-Up award at International Conference on Computational Photography 2015. He has served as an editorial board member of IJCV and an area chair of CVPR. Please access Camera Intelligence website for more information: http://ci.idm.pku.edu.cn